LLM Applications in Finance & Investing
Sentiment Analysis, Forecasting, Anomaly Detection, Agents & More
👋 Hi, I’m Andre and welcome to my newsletter Data-driven VC which is all about becoming a better investor with data & AI. Every Tuesday, I publish “Insights” to digest the most relevant startup research & reports, and every Thursday, I publish “Essays” that cover hands-on insights about data-driven innovation & AI in VC. Follow along to understand how startup investing becomes more data-driven, why it matters, and what it means for you.
Current subscribers: 24,305, +170 since last week
Brought to you by Affinity - Deal Sourcing Strategies That Set Top Firms Apart
Affinity’s 2024 investment benchmark report revealed that top VCs provide consistent engagement and invest in existing connections. The result? First access to the best deals. Learn how to put this strategy into action with Affinity on July 11. The webinar features insights and advice from Episode 1 Ventures, Illuminate Financial, and Dealroom.
Per our Data-Driven VC Landscape 2024, 2 out of 3 DDVC firms already use LLMs across their value chain. While our survey included some high-level applications such as Screening and Due Diligence, Sourcing, or Reporting, many of you asked for more specific use cases.
Today, I’m really excited to share a paper that I came across last night, providing a great overview of more detailed LLM applications across Finance and Investing. It’s written by a group of researchers from Princeton (Yuqi Nie, John M. Mulvey, H. Vincent Poor), Oxford (Yaxuan Kong, Xiaowen Dong, Stefan Zohren), and Squirrel AI (Qingsong Wen).
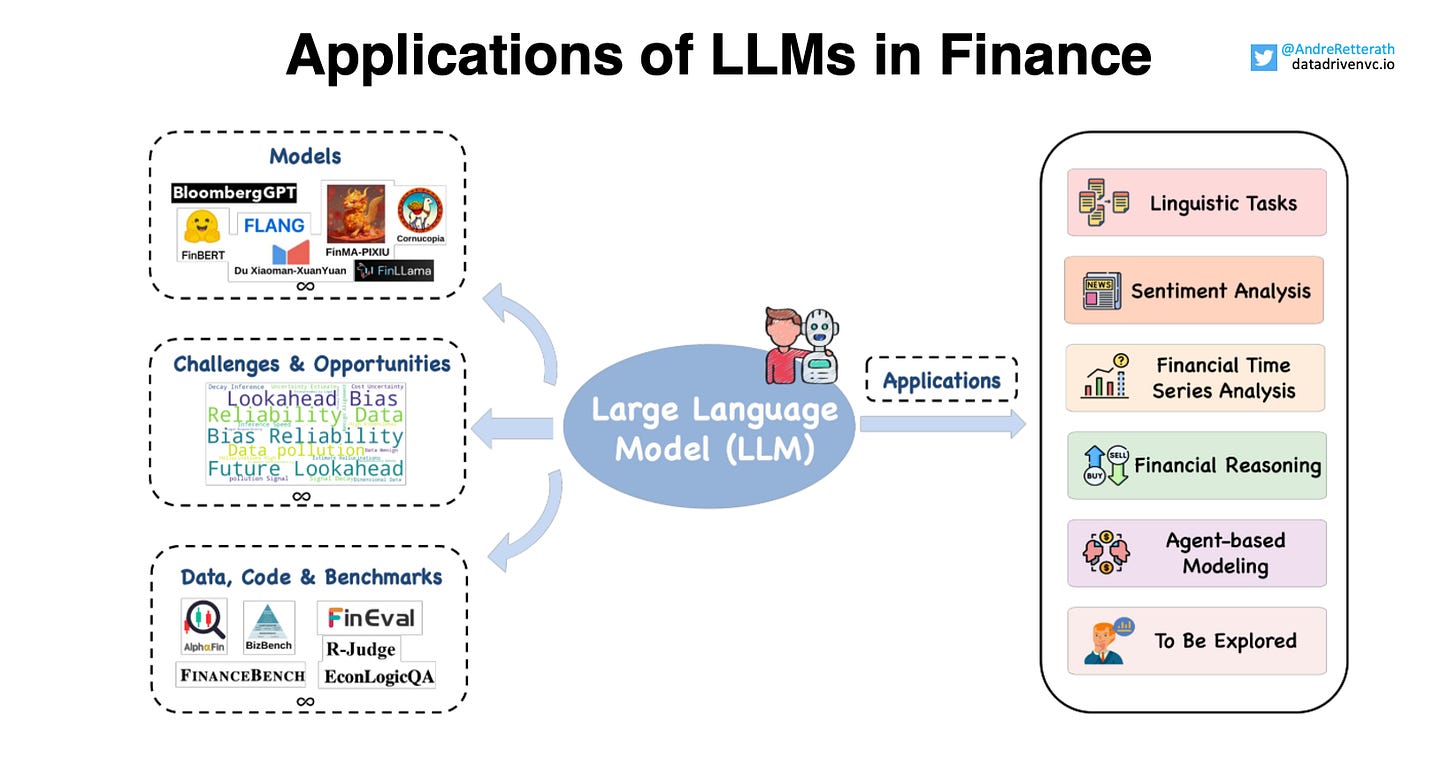
Overview of LLMs Focused on Finance
General-domain LLMs like GPT-series, Llama-series, and BERT have performed impressively on numerous NLP tasks, leading to the development of financial domain-specific LLMs. These specialized models are trained on extensive financial data, enhancing their ability to understand and generate finance-related content.
Among the notable financial LLMs is Ploutos, derived from GPT-4, designed for interpretable stock movement prediction. Ploutos integrates multimodal data and expert analysis to generate quantitative strategies and accurate stock predictions, despite challenges like expert selection bias and computational complexity.
BERT revolutionized NLP with its deep bidirectional architecture, and its financial variants like FinBERT-19, FinBERT-20, and FinBERT-21 have been particularly effective in sentiment analysis and financial text mining. Another variant, Mengzi-BERTbase-fin, focuses on financial applications using 20GB of financial news and research reports.
Google’s T5 model, with its text-to-text framework, has also seen a financial adaptation in BBT-FinT5, which is tailored for the Chinese financial sector. This model excels in language understanding and generation tasks within finance but may be limited outside this domain.
ELECTRA introduced a generator-discriminator framework for efficient language model training, leading to FLANG, a financial-specific variant excelling in sentiment analysis and entity recognition. BLOOM, a multilingual LLM, has inspired financial adaptations like BloombergGPT and XuanYuan 2.0, which offer enhanced performance in financial tasks and maintain competence across general language tasks. Llama-series models, including financial variants like InvestLM and FinGPT, offer specialized capabilities for financial applications, with InvestLM providing investment recommendations comparable to commercial models.
Meta’s Llama 3, introduced in 2024, features state-of-the-art performance and reasoning capabilities, with expectations for more financial variants. Other financial LLMs, such as FinTral, SilverSight, DISC-FinLLM, CFLLM, and FinVIS-GPT, leverage vast financial datasets and advanced training techniques for accurate financial analysis.
These models are transforming sectors like investment strategies, risk management, forecasting, and customer service. However, it's important to recognize their limitations and biases, and to use them in conjunction with human expertise and judgment to ensure effective financial decision-making.
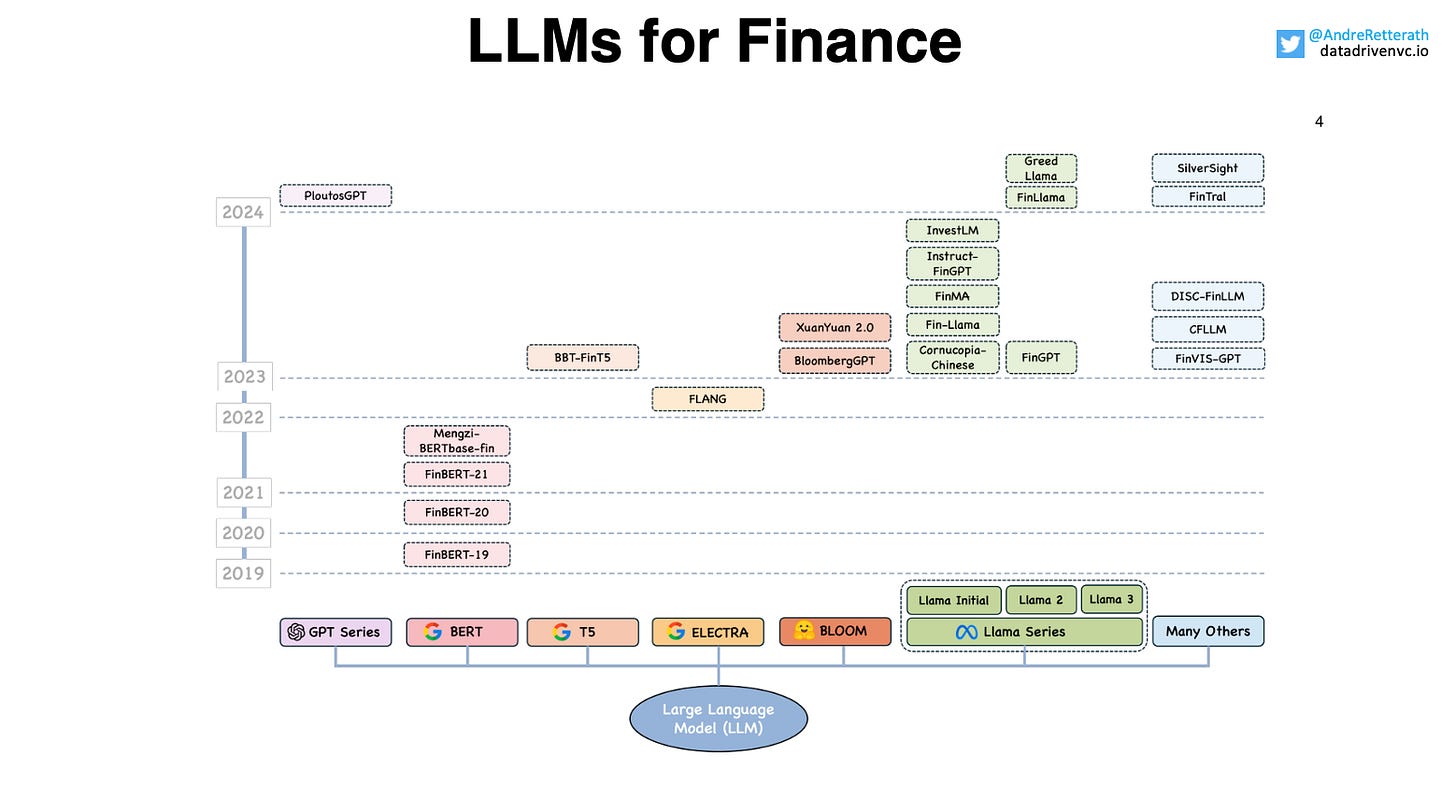