Data-driven VC #8: 3 steps to convince the data-skeptics/dinosaurs
Where venture capital and data intersect. Every week.
👋 Hi, I’m Andre and welcome to my weekly newsletter, Data-driven VC. Every Thursday I cover hands-on insights into data-driven innovation in venture capital and connect the dots between the latest research, reviews of novel tools and datasets, deep dives into various VC tech stacks, interviews with experts and the implications for all stakeholders. Follow along to understand how data-driven approaches change the game, why it matters, and what it means for you.
Current subscribers: 2,400, +313 since last week
Tl;dr
Convince the “VC is more art than science and impossible to automate”-skeptics with a simple but powerful 3-step approach
#1 “Inception”: Defeat initial skepticism with an objective benchmarking study; my “Human versus computer benchmarking study” shows that a basic XGBoost classification model predicts startup success at least as well as the best VC, 25% relatively better than the median VC and 29% relatively better than the average VC in our sample of 111 investors
#2 “Acceleration”: Deliver short-term ROI by creating strategic value for (new) LPs which then invest (more) money into your fund; the additional management fee justifies investments into a data platform and an engineering team
#3 “Final”: Deliver long-term ROI by a) identifying outlier opportunities that deliver superior returns for LPs and b) gaining a competitive edge towards founders that provides preferred access to the outlier opportunities; I show different ways of how to measure these dimensions
VC as an industry started in the 1940s and has seen little change ever since. The only visible progress was the move from pen and paper to computers, Excel and Powerpoint, as well as the recent shift from in-person meetings to more virtual meetings (at least for intro meetings; less for deep dive due diligence and team assessments) driven by COVID. That’s it. No other change, innovation or whatsoever. Isn’t it confusing that those who back the most disruptive entrepreneurs themselves still work like 80 years ago?
VCs historically refused to innovate
Trying to understand the root cause of innovation blockers in VC, I spoke to 150+ senior investors globally in the past 5 years. The spectrum of answers is wide, however, one single argument is front and center: “VC is more art than science. It is a human business that cannot be automated”. I agree but at the same time disagree. Yes, some parts of the value creation are more art than science (such as the final investment decision, looking each other in the eyes and deciding to partner together), but still, there are several components that are more science than art and can easily be automated (such as the data collection, identification, enrichment or screening).
I’ve walked you through my thinking on data collection, entity matching and feature engineering in previous episodes. There is really no need to explain why computers can do better than humans in these tasks. On the screening, however, skeptics are hard to convince and this is really where the above-mentioned statement is rooted. I framed this blocker as the “Automation-Control Trade-off” in the previous episode.
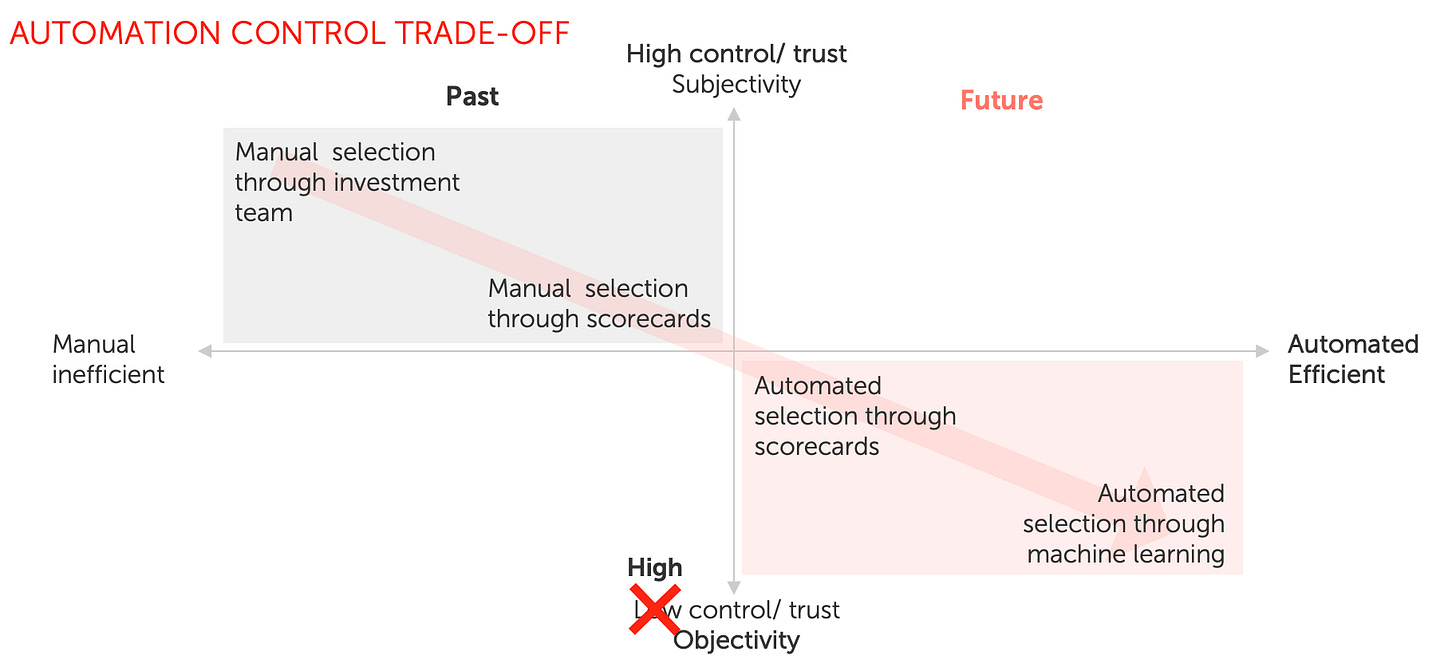
To solve this initial friction (=”Automation-Control Trade-off”) and justify subsequent investments into a holistic data-driven platform that is developed and maintained by an expert engineering team, I established a 3-staged strategy to convince the data-skeptics/dinosaurs.
Step#1 “Inception”: Solving the “Automation-Control Trade-off”
Considering the asymmetric cost matrix in screening (where false negatives are more costly than false positives; NOTE: it’s different for the final decision and we only focus on the screening here), VCs simply do not trust algorithms when it comes to the most critical part of their investment process.
To help resolve this trade-off and to allow the VC investment process to scale, I trained several ML-based screening algorithms, selected the best-performing one based on recall rate (inverse of false negative rate) and transparently compared its screening performance to the performance of human investment professionals. Why? Well, it’s hard to argue against data 🤓
Summary of “Human versus computer: Who’s the better startup investor?” benchmarking study
Purpose: Compare startup investment screening performance between ML-based algorithms and human investors
Metrics: Accuracy (AC) and recall (RE); the higher AC and RE, the better
Data sources: Based on our database benchmarking study (must read when working with startup data), I selected Crunchbase as the basis and matched it with Pitchbook and LinkedIn information
Test sample: 10 European software startups with anonymized input info right after they raised their Seed round in 2015/2016; 5 successful and 5 unsuccessful as of 2020
VC respondents: 111 investors
ML training sample: 77,279 European software startups, founded after 01.01.2010, run by 118,231 verified founders
ML algorithms (see Table 4 for comparison): decision trees (DT), random forests (RFs), gradient-boosted trees (also known as “XGBoost” (XG)), naive Bayes (NB), deep learning (DL) models, generalized linear (GL) models and logistic regressions (LRs)